Introduction
In the realm of statistical analysis, hypothesis testing plays a crucial role in validating research findings. The hypothesis testing results are often reported in the fourth chapter of the dissertation. Among the various statistical tests available, the one-sample t-test stands out as a powerful tool for researchers across diverse fields. The one-sample t-test allows scientists to compare a sample mean to a known or hypothesized population mean, making it an essential technique in many research scenarios.
The importance of the one-sample t-test in research cannot be overstated. This statistical method enables researchers to determine whether a sample’s mean significantly differs from a specified value, providing valuable insights into population characteristics. By employing the one-sample t-test, investigators can draw meaningful conclusions about their data and contribute to the broader scientific understanding of various phenomena.
This article aims to provide a comprehensive guide on conducting a one-sample t-test using SPSS (Statistical Package for the Social Sciences). We will explore the theoretical foundations of the one-sample t-test, its practical applications, and step-by-step instructions for performing this analysis in SPSS. By the end of this article, readers will have a solid understanding of when and how to use the one-sample t-test, as well as the ability to interpret and report its results accurately. Whether you’re a student, researcher, or data analyst, mastering the one-sample t-test will enhance your statistical toolkit and empower you to make data-driven decisions with confidence.
Hire SPSS Expert
Transform your data into meaningful insights with Best Dissertation Writers. Our SPSS experts provide accurate analysis, timely delivery, and detailed reports. Entrust your statistics assignments to us and achieve academic excellence. Place your order now!
Understanding the One-Sample T-Test
The one-sample t-test is a statistical procedure used to determine whether the mean of a sample significantly differs from a known or hypothesized population mean. This test is particularly useful when we have a single sample of data and want to compare it to a specific value or standard.
Definition and purpose:
The one-sample t-test is designed to assess whether the mean of a sample is statistically different from a specified value. It helps researchers answer questions like “Is the average score of students in our class significantly different from the national average?” or “Does the mean weight of products from our factory differ from the advertised weight?”
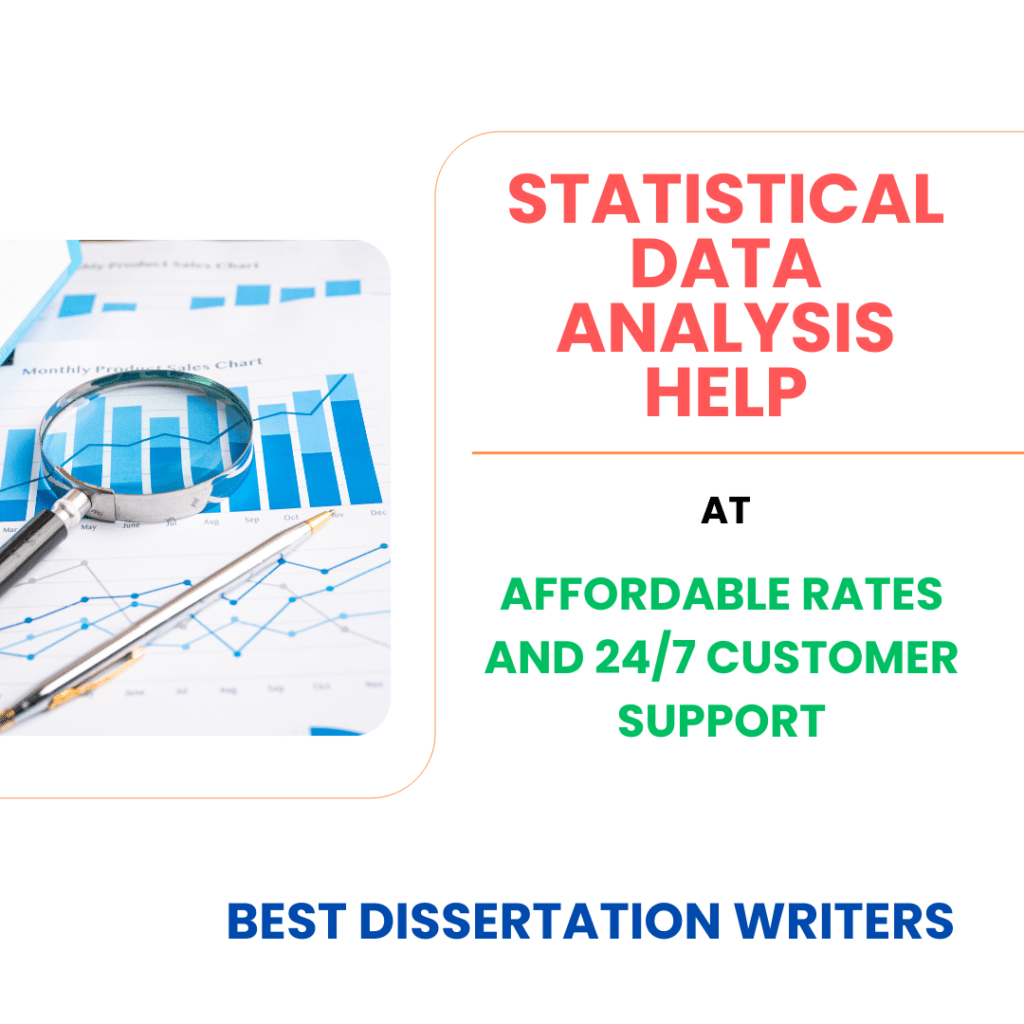
Assumptions of the test:
To ensure the validity of the one-sample t-test, certain assumptions must be met:
- The data should be normally distributed or approximately normal.
- The observations should be independent of each other.
- The variable being tested should be continuous or ordinal.
When to use a one-sample t-test:
The one-sample t-test is appropriate in various scenarios, including:
- Comparing a sample mean to a known population mean
- Testing whether a sample mean differs from a theoretical or hypothesized value
- Evaluating the effectiveness of an intervention by comparing pre- and post-test scores to a standard
Null and alternative hypotheses:
In a one-sample t-test, the null hypothesis (H0) typically states that there is no significant difference between the sample mean and the specified value. The alternative hypothesis (H1) suggests that there is a significant difference. These hypotheses can be expressed as:
H0: μ = μ0 (The sample mean is equal to the specified value)
H1: μ ≠ μ0 (The sample mean is not equal to the specified value)
Understanding these fundamental aspects of the one-sample t-test is crucial for its proper application and interpretation in research settings.
Preparing Data for Analysis in SPSS
Before conducting a one-sample t-test in SPSS, it’s essential to properly prepare your data. This preparation ensures accurate results and smooth analysis. Here are the key steps to prepare your data for a one-sample t-test in SPSS:
Data structure requirements:
For a one-sample t-test, your data should be organized in a single column in SPSS. Each row represents an individual observation or participant. Ensure that your variable is continuous or ordinal, as the one-sample t-test is not appropriate for categorical data.
Entering data into SPSS:
- Open SPSS and create a new data file.
- In the Variable View, define your variable by giving it a name, type (typically Numeric), and label.
- Switch to the Data View and enter your data into the column.
- If you have multiple variables, enter each in a separate column.
Checking data integrity:
Before proceeding with the one-sample t-test, it’s crucial to check your data for any errors or inconsistencies:
- Use SPSS’s Descriptive Statistics function to check for outliers or impossible values.
- Verify that there are no missing data points, or decide how to handle them if present.
- Check the normality assumption using histograms or normality tests like Shapiro-Wilk.
By carefully preparing your data and ensuring its integrity, you set a solid foundation for conducting an accurate one-sample t-test in SPSS. This preparation step is vital for obtaining reliable results and making valid inferences from your analysis.
Step-by-Step Guide to Conducting a One-Sample T-Test in SPSS
Conducting a one-sample t-test in SPSS is a straightforward process when you follow these step-by-step instructions. This guide will walk you through the entire procedure, from accessing the one-sample t-test function to running the analysis and interpreting the results.
Accessing the one-sample t-test function:
- Open your SPSS data file containing the variable you want to analyze.
- Click on “Analyze” in the top menu.
- Navigate to “Compare Means” and select “One-Sample T Test” from the dropdown menu.
Selecting variables:
- In the “One-Sample T-Test” dialog box, you’ll see a list of all variables in your dataset on the left side.
- Select the variable you want to analyze for the one-sample t-test.
- Click the arrow button to move the selected variable to the “Test Variable(s)” box on the right.
Setting the test value:
- In the “Test Value” field, enter the value you want to compare your sample mean against. This could be a known population mean, a theoretical value, or any other reference point relevant to your research question.
- Ensure that you enter a numeric value in this field.
Choosing confidence intervals:
- Click on the “Options” button in the “One-Sample T Test” dialog box.
- In the “Options” dialog, you can specify the confidence interval percentage. The default is usually 95%, but you can adjust this based on your research requirements.
- You can also choose to exclude cases listwise or pairwise if you have missing data.
Running the analysis:
- After setting all the parameters, click “OK” in the main “One-Sample T Test” dialog box.
- SPSS will now perform the one-sample t-test and generate the output in a new window.
Interpreting the SPSS Output:
Once SPSS has run the one-sample t-test, you’ll see several tables in the output viewer. Let’s break down the key components:
- One-Sample Statistics Table:
- This table provides descriptive statistics for your variable, including the sample size (N), mean, standard deviation, and standard error of the mean.
- One-Sample Test Table:
- This is the main results table for the one-sample t-test.
- It includes the t-statistic, degrees of freedom (df), significance level (Sig. 2-tailed), mean difference, and confidence interval of the difference.
- Interpreting the results:
- Look at the “Sig. (2-tailed)” value. This is your p-value.
- If the p-value is less than your chosen significance level (typically 0.05), you reject the null hypothesis and conclude that there is a significant difference between your sample mean and the test value.
- The “Mean Difference” shows how far your sample mean is from the test value.
- The “Confidence Interval of the Difference” provides a range within which the true population mean difference is likely to fall.
- Effect size:
- SPSS doesn’t automatically provide an effect size for the one-sample t-test.
- You can calculate Cohen’s d manually using the formula: d = (sample mean – test value) / standard deviation
By following these steps, you can successfully conduct a one-sample t-test in SPSS and interpret its results. Remember that the one-sample t-test is a powerful tool for comparing a sample mean to a known or hypothesized value, but always consider the assumptions and limitations of the test when drawing conclusions from your analysis.
SPSS Statistics Help
Unlock precise SPSS analysis for your projects with Best Dissertation Writers. Our seasoned statisticians deliver accurate results and comprehensive reports on time. Enhance your research quality by placing your statistics assignments with us today!
Practical Examples of One-Sample t-Test
Example 1: Testing Against a Known Population Mean
Scenario: A nutritionist wants to test if a new diet plan affects the average daily intake of calories. The average daily calorie intake for the population is known to be 2,500 calories. The nutritionist collects a sample of 30 individuals who followed the new diet plan for a month and records their daily calorie intake.
Hypothesis:
- Null Hypothesis (H0): The mean daily calorie intake of the sample is equal to the population mean of 2,500 calories.
- Alternative Hypothesis (H1): The mean daily calorie intake of the sample is different from the population mean.
SPSS Output:
Descriptive Statistics
Descriptive Statistics
N Mean Std. Deviation Std. Error Mean
----------------------------------------------
30 2450.67 250.56 45.76
One-Sample Test
One-Sample Test
Test Value = 2500
t df Sig. (2-tailed) Mean Difference 95% Confidence Interval of the Difference
Lower Upper
-------------------------------------------------------------------------------------------------
Calories -1.08 29 .288 -49.33 -142.85 44.19
Interpretation:
- The mean daily calorie intake of the sample is 2,450.67 calories.
- The t-statistic is -1.08 with a p-value of .288, which is greater than the alpha level of .05.
- We fail to reject the null hypothesis, concluding that there is no significant difference between the sample mean and the known population mean of 2,500 calories.
Example 2: Comparing a Sample to a Theoretical Value
Scenario: A teacher believes that a new teaching method improves students’ test scores. Historically, the average test score in this subject is 75. The teacher collects scores from a sample of 25 students who were taught using the new method.
Hypothesis:
- Null Hypothesis (H0): The mean test score of the sample is equal to the theoretical value of 75.
- Alternative Hypothesis (H1): The mean test score of the sample is different from the theoretical value.
SPSS Output:
Descriptive Statistics
Descriptive Statistics
N Mean Std. Deviation Std. Error Mean
----------------------------------------------
25 78.64 8.24 1.65
One-Sample Test
One-Sample Test
Test Value = 75
t df Sig. (2-tailed) Mean Difference 95% Confidence Interval of the Difference
Lower Upper
-------------------------------------------------------------------------------------------------
Test Scores 2.21 24 .037 3.64 .23 7.05
Interpretation:
- The mean test score of the sample is 78.64.
- The t-statistic is 2.21 with a p-value of .037, which is less than the alpha level of .05.
- We reject the null hypothesis, concluding that there is a significant difference between the sample mean and the theoretical value of 75. This suggests that the new teaching method may have a positive effect on students’ test scores.
In both examples, the one-sample t-test allows us to compare the sample mean against a known or theoretical value to determine if there is a statistically significant difference. This statistical test is valuable for validating hypotheses in various fields, from nutrition to education, providing insights into the effectiveness of new interventions or methods.
Reporting One-Sample T-Test Results
APA Format for Reporting t-Test Results
When reporting t-test results in APA format, it is crucial to include specific statistical details to ensure clarity and precision. The following elements should be included: the t-value, degrees of freedom (df), p-value, and the mean difference, along with confidence intervals if possible.
For example, the results from Example 1 (Testing Against a Known Population Mean) would be reported as:
- “A one-sample t-test was conducted to compare the daily calorie intake in the sample to the known population mean of 2,500 calories. There was no significant difference in the scores for the sample (M = 2450.67, SD = 250.56) and the population mean of 2,500, t(29) = -1.08, p = .288.”
For Example 2 (Comparing a Sample to a Theoretical Value), the reporting would be:
- “A one-sample t-test was conducted to compare the test scores of students taught with a new method to the theoretical mean of 75. There was a significant difference in the scores for the sample (M = 78.64, SD = 8.24) and the theoretical mean of 75, t(24) = 2.21, p = .037.”
Interpreting p-Values
The p-value in hypothesis testing indicates the probability of obtaining results at least as extreme as the observed results, assuming that the null hypothesis is true. It helps determine the significance of the results. Commonly, a p-value threshold (alpha level) of 0.05 is used.
- p-value < 0.05: The results are statistically significant, and the null hypothesis is rejected.
- p-value > 0.05: The results are not statistically significant, and there is insufficient evidence to reject the null hypothesis.
In Example 1, the p-value of .288 indicates that there is no significant difference between the sample mean and the population mean. In Example 2, the p-value of .037 suggests a significant difference between the sample mean and the theoretical value.
Drawing Conclusions
Drawing conclusions from t-test results involves interpreting the statistical significance and practical implications.
For Example 1, since the p-value is greater than 0.05, we conclude that the new diet plan does not significantly change the average daily calorie intake compared to the population mean. This suggests that the diet plan may not have a substantial impact on calorie intake.
In Example 2, the p-value is less than 0.05, indicating a significant difference between the sample mean test scores and the theoretical mean. This implies that the new teaching method may effectively improve student test scores.
When reporting conclusions, it is essential to consider both statistical significance and practical relevance to provide a comprehensive understanding of the results.
Dissertation Help Online
Best Dissertation Writers provides exceptional SPSS analysis for your assignments. Our expert team ensures accuracy, prompt delivery, and thorough interpretation. Rely on us for your statistical needs and achieve top results. Place your order today!
Template for Reporting One-Sample t-Test Results in APA Format
When reporting the results of a one-sample t-test in APA format, your results should include all of these sections. (1) context or introduction which provides an overview of the type of test conducted, (2) descriptive statistics sentence which report results from the descriptive analysis, (3) Hypothesis statement which outlines both the null and alternative hypotheses of the test, the one-sample t-test results outlining the outcomes from the t-test analysis and (4) conclusion statement providing a summary and meaning of results reported in relation to the tested hypothesis. You can always include all these sections in a single paragraph or two when reporting your results from one-sample t-test analysis.
Example Template:
Context/Introduction:
“A one-sample t-test was conducted to determine whether [insert variable, e.g., ‘the average test score’] of [insert sample description, e.g., ‘students in a new educational program’] was significantly different from [insert population mean or theoretical value, e.g., ‘the known population mean of 75’].”
Descriptive Statistics:
“Descriptive statistics showed that the sample had a mean score of M = [insert sample mean], with a standard deviation of SD = [insert standard deviation], and a standard error of the mean of SEM = [insert standard error].”
Hypothesis:
“The null hypothesis stated that the mean score of the sample would be equal to the population mean (H0: μ = [insert population mean]). The alternative hypothesis stated that the mean score would be different from the population mean (H1: μ ≠ [insert population mean]).”
One-Sample t-Test Results:
“A one-sample t-test indicated that there was [no significant/significant] difference between the sample mean (M = [insert sample mean], SD = [insert standard deviation]) and the population mean of [insert population mean], t([insert degrees of freedom]) = [insert t-value], p = [insert p-value], 95% CI ([insert lower confidence interval], [insert upper confidence interval]).”
Conclusion sentence:
“Based on these results, we [fail to reject/reject] the null hypothesis. This suggests that [insert conclusion based on the results, e.g., ‘the new educational program does not significantly affect the average test score compared to the known population mean’]. Further research might explore [insert suggestions for future research or implications of the findings].”
Example Using the Template
Context/Introduction:
“A one-sample t-test was conducted to determine whether the average test score of students in a new educational program was significantly different from the known population mean of 75.”
Descriptive Statistics:
“Descriptive statistics showed that the sample had a mean score of M = 78.64, with a standard deviation of SD = 8.24, and a standard error of the mean of SEM = 1.65.”
Hypothesis:
“The null hypothesis stated that the mean score of the sample would be equal to the population mean (H0: μ = 75). The alternative hypothesis stated that the mean score would be different from the population mean (H1: μ ≠ 75).”
One-Sample t-Test Results:
“A one-sample t-test indicated that there was a significant difference between the sample mean (M = 78.64, SD = 8.24) and the population mean of 75, t(24) = 2.21, p = .037, 95% CI (0.23, 7.05).”
Conclusion sentence:
“Based on these results, we reject the null hypothesis. This suggests that the new educational program significantly affects the average test score compared to the known population mean. Further research might explore the specific aspects of the program that contribute to this improvement in scores.”
By following this template, you can ensure that your one-sample t-test results are reported clearly and comprehensively in APA format.
Alternatives to the One-Sample t-Test
Non-Parametric Alternatives
When the assumptions of the one-sample t-test, such as normality of the data, are not met, non-parametric alternatives can be used. The Wilcoxon Signed-Rank Test is a common non-parametric test that compares the median of a single sample to a specified value. This test is particularly useful for small sample sizes or when the data is skewed or ordinal.
When to Use Other Tests
- Paired t-Test: Use this test when comparing two related samples, such as measurements before and after a treatment on the same subjects. It assesses whether the mean difference between paired observations is zero.
- Independent t-Test: This test compares the means of two independent groups. It is suitable when you have two separate groups and want to determine if their means are significantly different from each other.
Choosing the appropriate test depends on the data structure and the specific research questions being addressed.
Common Pitfalls in Conducting One-Sample T-Tests and How to Avoid Them
Violating Assumptions
One of the most common pitfalls in conducting a one-sample t-test is violating its underlying assumptions. The primary assumptions include the normality of the data and the scale of measurement being continuous. To avoid violating these assumptions:
- Check for Normality: Use visual inspections (e.g., Q-Q plots) and statistical tests (e.g., Shapiro-Wilk test) to assess the normality of your data. If the data is not normally distributed, consider using a non-parametric alternative like the Wilcoxon Signed-Rank Test.
- Appropriate Scale: Ensure that the data is continuous and measured on an interval or ratio scale. For ordinal or nominal data, non-parametric tests are more suitable.
Misinterpreting Results
Misinterpreting the results is another common pitfall. A significant t-test result indicates that the sample mean is statistically different from the test value, but it does not imply practical significance or causality. To avoid misinterpretation:
- Contextualize Results: Consider the practical significance of your findings and the effect size. Statistical significance does not always equate to practical importance.
- Avoid Overgeneralization: Be cautious about generalizing results beyond the scope of the study sample and context. The findings are valid for the specific sample and conditions under which the data was collected.
Overreliance on p-Values
Overreliance on p-values can lead to misinterpretation and overemphasis on statistical significance. A p-value indicates the probability of observing the data assuming the null hypothesis is true, but it does not measure the size of an effect or its importance. To avoid overreliance:
- Report Effect Sizes: Alongside p-values, report effect sizes (e.g., Cohen’s d) to provide a sense of the magnitude of the observed effect.
- Consider Confidence Intervals: Confidence intervals offer insight into the precision of the estimated effect and the range within which the true effect likely falls.
- Holistic Interpretation: Use p-values as one piece of evidence in a broader context, considering other factors such as study design, data quality, and theoretical implications.
By being mindful of these pitfalls and employing a comprehensive approach to data analysis, researchers can ensure more robust and meaningful interpretations of their one-sample t-test results.
Hypothesis Testing Help
Elevate your statistics projects with Best Dissertation Writers. Our SPSS specialists offer meticulous analysis, fast turnaround, and insightful reports. Trust us for your data analysis needs and excel in your studies. Order now!
Conclusion
In summary, the one-sample t-test is a valuable tool for comparing a sample mean to a known or theoretical value, provided its assumptions are met. Proper application and interpretation are crucial, involving checking assumptions, contextualizing results, and reporting effect sizes alongside p-values. Avoiding common pitfalls such as violating assumptions and overreliance on p-values ensures robust analysis. As statistical methods advance, incorporating more sophisticated techniques and non-parametric alternatives can enhance data analysis, offering deeper insights and more accurate conclusions. Future directions in statistical analysis will likely emphasize the integration of these advanced methodologies to improve research quality.