What is a Simple Random Sample?
Definition of a Simple Random Sample
Simple random sampling is one of the most fundamental probability sampling methods used in research and statistical analysis. As a type of probability sampling, it forms the foundation for many research methodologies. This comprehensive overview of simple random sampling provides definition and examples to help researchers make informed decisions about their sampling strategy.
Simple random sampling is a type of sampling where each member of the population has an equal chance of being selected for inclusion in the study. In this method, sampling involves collecting data through a process where every individual or item in the population has an equal chance of being chosen. Simple random sampling is a technique that ensures unbiased selection, making it one of the most reliable sampling designs available to researchers.
When a researcher uses simple random sampling, they ensure that each member of the population has an equal probability of being selected. This random selection may occur through various methods, but the key principle remains that sampling involves choosing elements without any predetermined pattern or bias.
Struggling with your dissertation? Let Best Dissertation Writers guide you to success. Our experienced academic writers provide tailored support, in-depth research assistance, and expert editing services. Request your personalized dissertation plan today.
How Does Simple Random Sampling Work?
Random sampling involves collecting data by selecting participants where each element has an equal chance of being selected. The process relies on random selection through either computerized methods or manual techniques. Simple random sampling allows researchers to obtain a representative sample of the larger population without systematic bias.
For example, a random sample would be created when selecting 100 students from a university of 10,000 students, where every student has an equal chance of being chosen. This sampling strategy ensures that the selection process remains fair and unbiased.
Characteristics of a Simple Random Sample
- Each element has an equal probability of being selected
- The selection process creates a random subset of the entire population
- Sampling occurs within the defined population boundaries
- Random selection may be conducted using various tools
- Every member of the population has an equal chance of being included in the sample
- Simple random sampling allows for statistical inference
- The method provides a foundation for other probability sampling methods
What are the Advantages and Disadvantages of Simple Random Sampling?
Advantages of the Simple Random Sampling Method
- High representativeness when properly executed
- Simple random sampling allows for straightforward statistical analysis
- Minimal knowledge of the population required beforehand
- Sampling error can be easily calculated
- Selection bias is minimized due to the random nature of selection
- Simple random sampling techniques provide reliable data
- The method allows for valid statistical inference
- Random samples are used to make predictions about the larger population
Disadvantages of Simple Random Sampling
Among the disadvantages of this method are:
- Requires a complete sample frame listing all population members
- Can be time-consuming for large populations
- May be expensive to implement
- Simple random sampling may not capture specific subgroups adequately
- Random sampling involves potential logistical challenges
- Sampling may result in underrepresentation of minority groups
- The selection method might be impractical for geographically dispersed populations
When to Use a Simple Random Sample
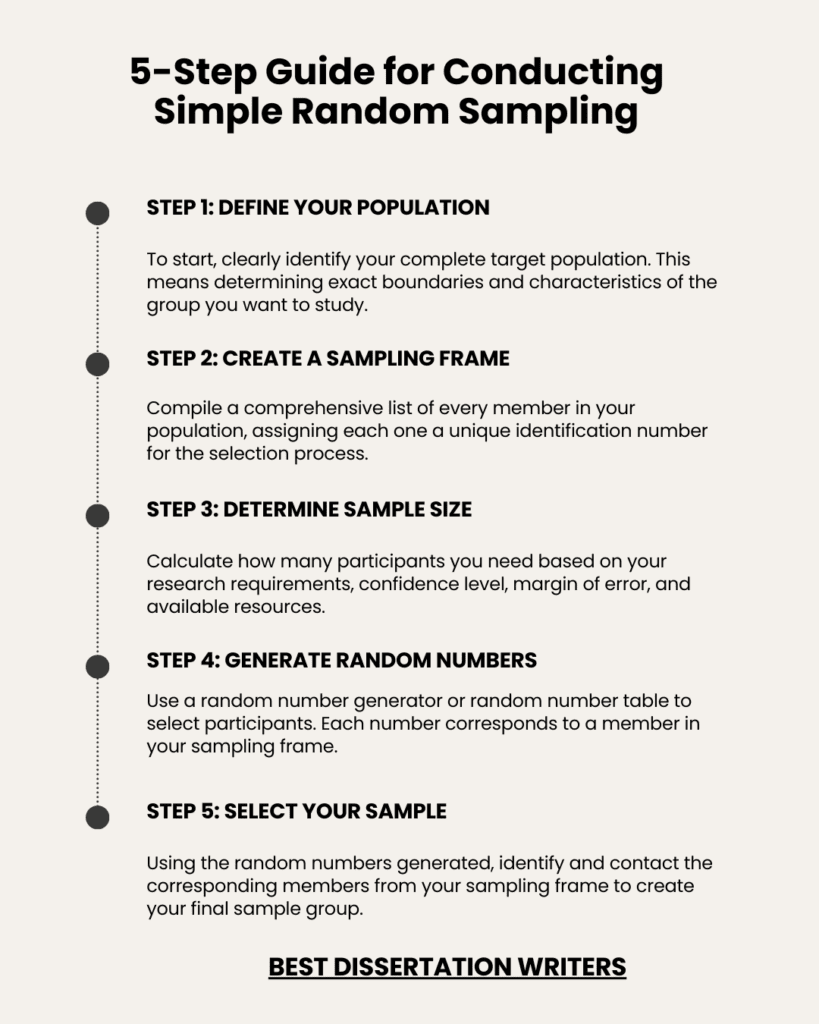
Researchers should use simple random sampling when:
- A representative sample is needed
- The population is relatively homogeneous
- A sample frame is readily available
- Statistical inference is required
- Resources allow for random selection of participants
- The entire population is accessible
- Simple random sampling techniques can be properly implemented
How to Create a Simple Random Sample?
Steps to Perform Simple Random Sampling
- Define the target population
- Create a comprehensive list of all members
- Assign unique numbers to each member
- Generate random numbers
- Select participants based on the random numbers
- Verify that sampling involves proper randomization
- Document the selection process
- Implement the sampling strategy
Using a Random Number Generator
A random number function in computer programs or calculators can help generate random numbers. This method is efficient and eliminates human bias in the selection process. When using random number generators:
- Ensure the generator produces truly random numbers
- Set appropriate ranges for your population size
- Document the seed number if applicable
- Verify the random selection process
- Keep records of the generated numbers
Utilizing the Random Number Table
To use a random number table:
- Establish a random starting point
- Move systematically through the table
- Select numbers that correspond to your population
- Continue until you reach your desired sample size
- Use a random number table consistently
- Document the selection process
- Maintain records of selected numbers
The lottery method is another traditional approach to random selection, though it’s less common in modern research.
Don’t let dissertation stress hold you back. Best Dissertation Writers offers expert guidance, comprehensive research support, and personalized writing assistance to help you achieve your academic goals. Contact us today to start your journey toward graduation success.
What are the Types of Random Sampling Techniques?
An Overview of 4 Types of Random Sampling
The four types of random sampling commonly used are:
- Simple random sampling
- Systematic sampling
- Stratified random sampling
- Cluster random sampling
Each of these probability sampling methods serves different research needs and population characteristics.
Differences Between Simple Random Sampling and Other Methods
- Systematic Random Sampling: Uses a fixed interval selection after a random starting point
- Systematic sampling follows a predetermined pattern
- Stratified Sampling: Divides population into strata before random selection
- Cluster Sampling: Groups population into clusters for efficiency
- Additional sampling methods may combine these approaches
- Two-stage cluster sampling often incorporates multiple techniques
- Each type of sampling has specific applications
When to Use Different Sampling Techniques
Different scenarios call for different sampling methods:
- Use simple random sampling for homogeneous populations
- Systematic random sampling when ordered lists exist
- Stratified sampling for distinct subgroups
- Cluster random sampling for geographically dispersed populations
- Consider population characteristics when selecting a method
- Evaluate resource constraints
- Match sampling design to research objectives
How Does Stratified Random Sampling Compare to Simple Random Sampling?
Definition and Process of Stratified Random Sampling
Stratified random sampling occurs within predetermined groups called strata. Like simple random sampling, it maintains randomness but within each stratum. This process ensures representation from all important subgroups in the final sample.
The key steps in stratified sampling include:
- Dividing the population into strata
- Sampling occurs within each stratum
- Combining results for analysis
- Ensuring proportional representation
Advantages of Stratified Sampling
- Better representation of subgroups
- Increased statistical precision
- Allows for analysis within strata
- Reduces overall sampling error
- More efficient than simple random sampling in heterogeneous populations
- Ensures minority groups are represented
- Provides more precise population estimates
When to Choose Stratified Random Sampling
Choose stratified sampling when:
Population has distinct subgroups
- Ensuring representation of minority groups is crucial
- Sampling involves collecting data from different demographic segments
- Comparison between groups is needed
- The population is highly diverse
- Precision is crucial for subgroup analysis
- Resources allow for more complex sampling
How to Determine Sample Size in Simple Random Sampling?
Factors Influencing Sample Size
Several factors affect the required sample size:
- Desired confidence level
- Margin of error
- Population variability
- Resource constraints
- Expected response rate
- Statistical power requirements
- Population characteristics
- Research objectives
Calculating the Sample Size
To calculate sample size:
- Determine the desired precision
- Consider the population variance
- Account for confidence level
- Adjust for potential non-response
- Evaluate practical constraints
- Use appropriate statistical formulas
- Consider resource limitations
- Document calculation methods
Impact of Sample Size on Sampling Bias
- Larger samples generally reduce sampling error
- Small samples may not be representative of the population
- Sample means become more reliable with larger samples
- Practical considerations must balance statistical requirements
- Random samples are used to minimize bias regardless of size
- Sample size affects confidence intervals
- Larger samples provide more precise estimates
- Cost considerations may limit sample size
Transform your research into a masterpiece with Best Dissertation Writers. Our team of PhD-qualified experts is ready to help you craft a compelling dissertation that meets the highest academic standards. Book your free consultation now!
Simple random sampling is a fundamental method of sampling that provides a solid foundation for statistical analysis. When a researcher uses simple random sampling, they ensure that every member of the population has an equal chance of being selected. While it has its limitations, understanding when and how to use simple random sampling techniques can help researchers select the most appropriate sampling method for their specific needs. Whether using random number tables or computerized methods, the key is ensuring that the selection process maintains the principle of equal probability of selection for all population members.
This overview of simple random sampling demonstrates its importance in research methodology and its relationship to other sampling designs. Researchers must carefully consider their specific needs and constraints when choosing between simple random sampling and other probability sampling methods to ensure the most effective research design.
FAQs about Simple Random Sampling
Which is an example of a simple random sample?
A classic example of simple random sampling is when conducting a school survey where researchers randomly select 100 students from a list of 1,000 students. For instance, selecting a random sample might involve assigning each student a number and using a list of random numbers to choose participants. Another example of simple random sampling could be when a company needs to select 50 products for quality control from 500 items on a production line. Sampling is a method that ensures each item has an equal chance of being chosen through a single random selection process.
What are the 4 types of random sampling?
When researchers need to select a sample for their survey, they can choose from four main types of random sampling methods. The first is simple random sampling, where participants are chosen using a list of random numbers. The second is systematic sampling, where researchers select every nth item after a random start. Third is stratified sampling, where the population is divided into subgroups before randomly selecting participants. Fourth is cluster sampling, where the population is divided into clusters, and entire clusters are randomly chosen. Each method suits different research needs.
Why is simple random sampling the best?
Simple random sampling is often considered superior because it provides the most unbiased way to select a sample. When researchers need to randomly select participants for a survey, this sampling is a method that gives every member of the population an equal chance of being chosen. An example of simple random sampling’s effectiveness is seen in large-scale public opinion polls. The process of selecting a random sample eliminates human bias and ensures statistical validity. Using a single random selection approach with a list of random numbers makes the results more representative and generalizable.
What are the pros and cons of a simple random sample?
The advantages of simple random sampling include its unbiased nature when researchers randomly select participants. As an example of simple random sampling, when conducting a survey, every member has an equal chance of being chosen. The method is straightforward: selecting a random sample using a list of random numbers ensures fairness. However, some disadvantages exist. It can be expensive and time-consuming to obtain a complete sampling frame, and the selected sample may not perfectly represent small subgroups in the population. Additionally, it may be logistically challenging when dealing with large, geographically dispersed populations.