What are the types of probability sampling methods?
Probability sampling methods form the cornerstone of reliable statistical research, providing researchers with systematic approaches to study populations effectively. When conducting research studies, understanding these different types of probability sampling is crucial as sampling allows researchers to make valid inferences about larger populations from manageable samples. Probability sampling is a sampling method that ensures every member of the population has a known chance of being selected, making it fundamental to scientific inquiry and data-driven decision-making.
Different types of sampling methods exist within the probability sampling framework, each serving specific research needs. Probability sampling is a sampling approach that’s firmly based on probability theory, ensuring that each member of a population has a known probability of being included in the study. The sampling process involves random selection to some degree, which helps eliminate systematic bias.
Different types of probability sampling include simple random sampling, stratified sampling, cluster sampling, and systematic sampling. Each of these sampling methods in statistics offers unique advantages and can be applied in various research contexts. When choosing between different sampling methods, researchers must consider their specific research objectives, resources, and the characteristics of their target population.
Transform your dissertation journey with Best Dissertation Writers‘ expert consultation services. Our experienced PhD mentors provide guidance on research methodology, literature review strategies, and academic writing techniques to help you excel independently.
What is a simple random sample?
Using simple random sampling represents the most fundamental of all probability sampling techniques. In this method, every member of the population has an equal chance of being selected for the study. When you use random sampling to select participants, each member of a population receives the same probability of selection, making it one of the most unbiased approaches available.
The sampling process for simple random sampling requires:
- A complete list of the target population
- A method for random selection
- Equal opportunity for every population member to be chosen
- No systematic exclusion of any population segments
Simple random sampling is particularly effective when:
- The population is relatively homogeneous
- You have a complete and accurate sampling frame
- You want to minimize selection bias
- Statistical simplicity is desired
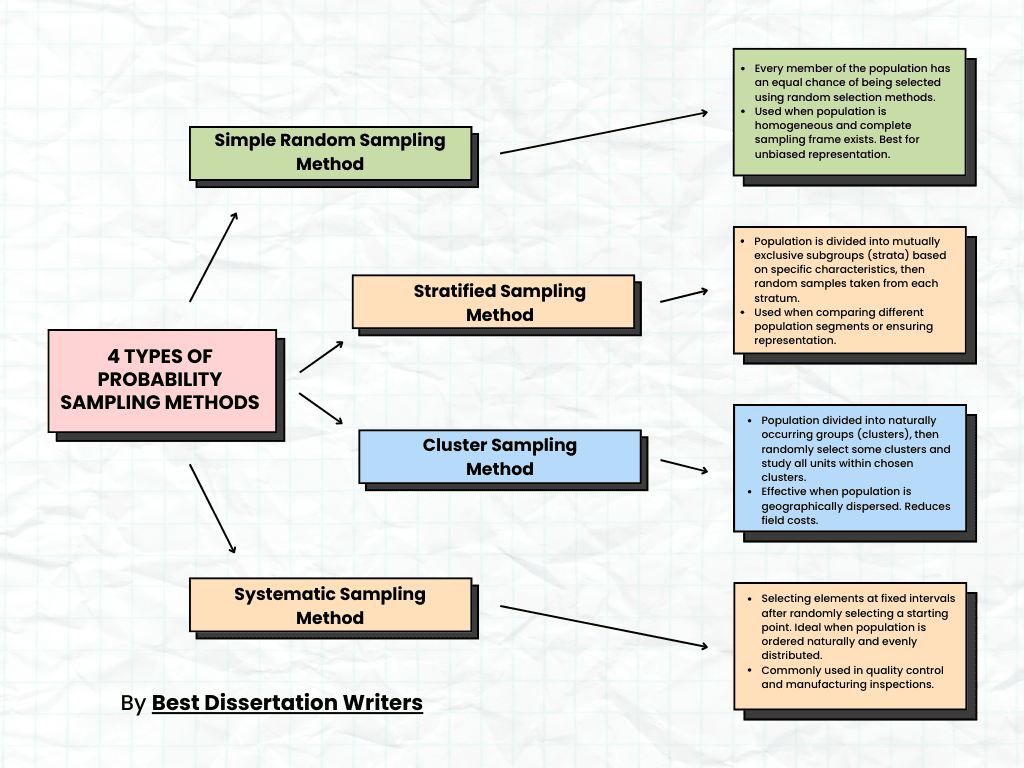
How does stratified sampling work?
Stratified sampling involves dividing the population into distinct subgroups (strata) based on specific characteristics before sampling. Like stratified sampling suggests, this approach ensures representation across all important population segments. Stratified sampling can ensure that even small but significant subgroups are included in the final sample, making it particularly valuable for heterogeneous populations.
The process of stratified random sampling includes:
- Identifying relevant stratification variables
- Dividing the population into strata
- Sampling from each stratum independently
- Combining results for final analysis
Stratified sampling involves considerations such as:
- Proportional vs. disproportional allocation
- Selection of stratification variables
- Sample size determination for each stratum
- Integration of results across strata
What is cluster sampling and when to use it?
Cluster sampling divides the population into naturally occurring groups or clusters, and sampling is similar to stratified sampling in some ways, but with key differences. When you use cluster sampling, you select entire clusters rather than individual members, making it particularly efficient for geographically dispersed populations. Multistage cluster sampling takes this approach further by implementing multiple levels of cluster selection.
Key aspects of cluster sampling include:
- Natural grouping of population elements
- Selection of entire clusters
- Potential for multistage sampling
- Cost-effective implementation
Multistage cluster sampling often involves:
- Primary sampling units (larger clusters)
- Secondary sampling units (sub-clusters)
- Final sampling units (individual elements)
- Progressive selection at each stage
What is the systematic sampling method?
When you use systematic sampling, you select members at regular intervals after a random start point. The sampling interval is calculated by dividing the total population size by the desired sample size. Systematic sampling to select participants can be particularly efficient when working with ordered lists or continuous processes. This sampling method is similar to simple random sampling but offers some practical advantages in implementation.
Key elements of systematic sampling include:
- Calculating the sampling interval
- Selecting a random starting point
- Following the interval pattern
- Maintaining selection consistency
Systematic sampling also involves:
- Regular spacing between selections
- Coverage of the entire population
- Potential for periodicity issues
- Simple implementation procedures
What are the advantages and disadvantages of probability sampling?
What are the advantages of probability sampling?
Probability sampling allows researchers to make valid statistical inferences about the whole population. Probability sampling gives researchers several key advantages:
Statistical Validity:
- Based on probability theory
- Allows for error estimation
- Supports hypothesis testing
- Enables confidence interval calculation
Representation:
- Representative of the population
- Reduces selection bias
- Supports generalizable findings
- Probability sampling can help ensure diversity
Scientific Rigor:
- Sampling allows for systematic research
- Supports replication studies
- Enables comparison across studies
- Maintains research integrity
Ready to strengthen your dissertation? Our professional editors offer comprehensive feedback on structure, clarity, and academic style while preserving your original work. Book a consultation to discuss your research goals.
What are the disadvantages of probability sampling?
While probability sampling techniques offer many benefits, probability sampling isn’t without its challenges:
Resource Demands:
- Higher costs
- Increased time requirements
- Need for expertise
- Complex implementation
Practical Challenges:
- Higher risk of sampling bias if poorly executed
- Need for complete sampling frame
- Potential for non-response issues
- Complex sampling design requirements
Implementation Issues:
- Risk of sampling error
- Sampling isn’t always feasible
- Requires strict protocol adherence
- May need specialized software
How does sampling error affect results?
Sampling error occurs because a sample may not perfectly represent the larger population from which it’s drawn. Understanding how sampling error affects research outcomes is crucial for:
Statistical Inference:
- Confidence interval calculation
- Margin of error determination
- Significance testing
- Result interpretation
Research Design:
- Sample size determination
- Power analysis
- Methodology selection
- Error minimization strategies
How do probability and non-probability sampling differ?
What is the difference between probability sampling and non-probability sampling?
The distinction between probability sampling and non-probability sampling methods is fundamental to research methodology. Methods include simple random sampling and various other approaches:
Probability Sampling Techniques:
Random Selection:
- Population has a known chance
- Equal chance of being selected
- Systematic selection procedures
- Mathematical probability basis
Statistical Inference:
- Sampling allows for generalization
- Error estimation possible
- Population parameters estimation
- Hypothesis testing capability
Non-probability sampling techniques include:
Convenience Methods:
- Convenience sample usage
- Quota sampling approaches
- Purposive sample selection
- Snowball sampling techniques
When should I use non-probability sampling methods?
While probability sampling allows for statistical inference, there are situations where sampling isn’t feasible using probability methods. Consider non-probability approaches when:
Research Context:
- Exploratory studies
- Pilot research
- Market research needs
- Qualitative investigations
Practical Constraints:
- Limited resources
- Time restrictions
- Access limitations
- Budget constraints
What are some examples of probability sampling?
Can you provide examples of simple random sampling?
Examples of where you might use random sampling to select participants include:
Academic Research:
- Student selection for surveys
- Course evaluation participants
- Research subject recruitment
- Laboratory experiment sampling
Business Applications:
- Quality control testing
- Employee satisfaction surveys
- Customer feedback collection
- Product testing groups
What are real-world applications of stratified sampling?
Stratified sampling involves dividing populations in various contexts:
Market Research:
- Consumer behavior studies
- Product preference analysis
- Brand awareness surveys
- Customer satisfaction research
Social Research:
- Demographic studies
- Opinion polls
- Educational assessments
- Healthcare surveys
How is cluster sampling utilized in research?
When you use cluster sampling, applications typically include:
Geographic Studies:
- Regional health surveys
- Educational district assessment
- Urban planning research
- Environmental sampling
Organizational Research:
- Multi-site business studies
- Franchise performance analysis
- Employee satisfaction surveys
- Operational efficiency studies
How to use probability sampling effectively?
What are the best practices for sampling technique?
To make the sampling process effective, consider:
Planning Phase:
- Define target population clearly
- Choose right sampling method
- Develop sampling strategy
- Calculate appropriate sample size
Implementation:
- Follow sampling design strictly
- Monitor data collection
- Maintain selection protocols
- Document procedures thoroughly
Quality Control:
- Verify selection procedures
- Monitor response rates
- Check for sampling bias
- Validate data collection
Enhance your dissertation’s impact with our specialized academic editing services. Our team of field experts provides detailed feedback on methodology, analysis, and scholarly presentation while maintaining your authentic voice.
How to design a sampling plan?
Creating an effective sampling plan involves:
Initial Planning:
- Define research objectives
- Identify target population
- Select sampling frame
- Choose sampling approach
Technical Specifications:
- Determine sample size
- Specify selection procedures
- Establish sampling interval
- Define inclusion criteria
Implementation Guidelines:
- Create selection protocols
- Establish quality controls
- Define documentation requirements
- Plan for contingencies
What is the role of sample frame in sampling?
What is a sample frame and how is it created?
A sample frame serves as the foundation for selecting a sample from a population:
Development Process:
- Population identification
- Member listing
- Information verification
- Update procedures
Quality Considerations:
- Completeness check
- Accuracy verification
- Currency maintenance
- Accessibility assessment
Management Procedures:
- Regular updates
- Error correction
- Duplicate removal
- Coverage verification
How does a sample frame affect sampling bias?
The quality of your sample frame directly impacts the risk of sampling bias:
Coverage Issues:
- Population representation
- Inclusion/exclusion errors
- Frame accuracy
- Update frequency
Selection Problems:
- Access limitations
- Response patterns
- Sampling error sources
- Bias introduction points
Quality Impact:
- Research validity
- Result reliability
- Generalizability
- Statistical inference
Remember that sampling methods in research require careful attention to detail and systematic implementation. Whether you use this sampling approach or another, ensuring proper execution is crucial for valid results. The sampling method for your research should align with your objectives, resources, and population characteristics to optimize research outcomes.
FAQs about Probability Sampling Methods
What are the 4 types of probability sampling?
Probability sampling methods include simple random sampling, stratified sampling, systematic sampling, and cluster sampling. Sampling involves random selection in each type, but the approach differs. Simple random sampling gives every member an equal chance of selection. Stratified sampling divides the population into subgroups.
Systematic sampling is a sampling technique that selects members at fixed intervals. Cluster sampling is the process of dividing the population into groups and randomly selecting entire clusters. Sampling can also combine these methods in more complex designs like multistage cluster sampling for large-scale studies.
What is an example of a random probability sample?
Methods and examples of random probability sampling can be found in various contexts. Consider a high school with 1000 students where researchers need 100 participants for a study. Sampling is the process of assigning each student a number from 1-1000, then using a random number generator to select 100 numbers.
Each student has an equal chance of being chosen. Sampling can also be done using software or random selection tables. This ensures unbiased selection where every member of the population has the same probability of being included in the study.
What is the best probability sampling method?
The best probability sampling method depends on your research goals and population characteristics. Sampling methods include simple random sampling as the most basic and statistically robust approach. However, sampling can also be more effective using stratified sampling when the population has distinct subgroups that need representation.
Sampling involves random selection in all cases, but the “best” method balances statistical validity with practical constraints like cost, time, and accessibility. Each technique has unique advantages – the key is matching the method to your specific research needs.
What are the 5 basic sampling methods and examples?
The five basic sampling methods include both probability and non-probability approaches. Probability methods include: (1) Simple random sampling – selecting lottery winners, (2) Stratified sampling – dividing students by grade level, (3) Systematic sampling – selecting every 10th customer.
Non-probability methods include: (4) Convenience sampling – surveying available shoppers, and (5) Purposive sampling – choosing experts for interviews. Sampling is a sampling technique that must match research goals. Each method has specific applications where it works best, depending on research objectives and resources available.