What is Data Extraction Table in Systematic Reviews?
Understanding the Data Extraction Process
The data extraction table is a crucial tool in systematic reviews and meta-analyses. It serves as the foundation for collecting and organising information from primary studies. The process of data extraction is a key step in conducting a systematic review, where relevant information is systematically identified and recorded from included studies.
Data extraction is the process of methodically gathering and recording information from primary research studies that have been identified as meeting the inclusion criteria for a systematic review. This process typically involves using a standardised data extraction form or table to ensure consistency and completeness in data collection across all included studies.
Struggling with your nursing systematic review and dissertation writing? Let Best Dissertation Writers streamline your research process. We’ll help you synthesize evidence, analyze findings, and present a cohesive review. Take the first step towards academic excellence—contact us now.
Importance of Data Extraction Table in Systematic Reviews
The data extraction table plays a vital role in systematic reviews for several reasons:
- Standardisation: It provides a structured approach to collect data consistently across all included studies.
- Comprehensiveness: It ensures that all relevant data points are captured for each study.
- Transparency: It allows for clear documentation of the data extraction process, enhancing the reproducibility of the review.
- Efficiency: It streamlines the data collection process, making it easier for review authors to manage large amounts of information.
- Analysis preparation: The organised data facilitates subsequent analysis and synthesis of findings.
Key Terms Related to Data Extraction
To better understand the context of data extraction tables, it’s important to familiarise yourself with the following key terms:
- Systematic review: A comprehensive and structured analysis of existing research on a specific topic.
- Meta-analysis: A statistical method for combining results from multiple studies.
- Scoping review: A type of review that aims to map the available evidence on a topic.
- Data collection form: A structured template used to gather information from primary studies.
- Review team: The group of researchers involved in conducting the systematic review.
- Data extractors: Individuals responsible for extracting data from included studies.
- Cochrane: An international organisation that produces high-quality systematic reviews in healthcare.
- Cochrane Handbook: A comprehensive guide for conducting systematic reviews of interventions.
Understanding these terms is crucial for effectively using and creating data extraction tables in systematic reviews and meta-analyses.
How to Create an Effective Data Extraction Form?
Components of a Data Extraction Table
An effective data extraction table should include the following key components:
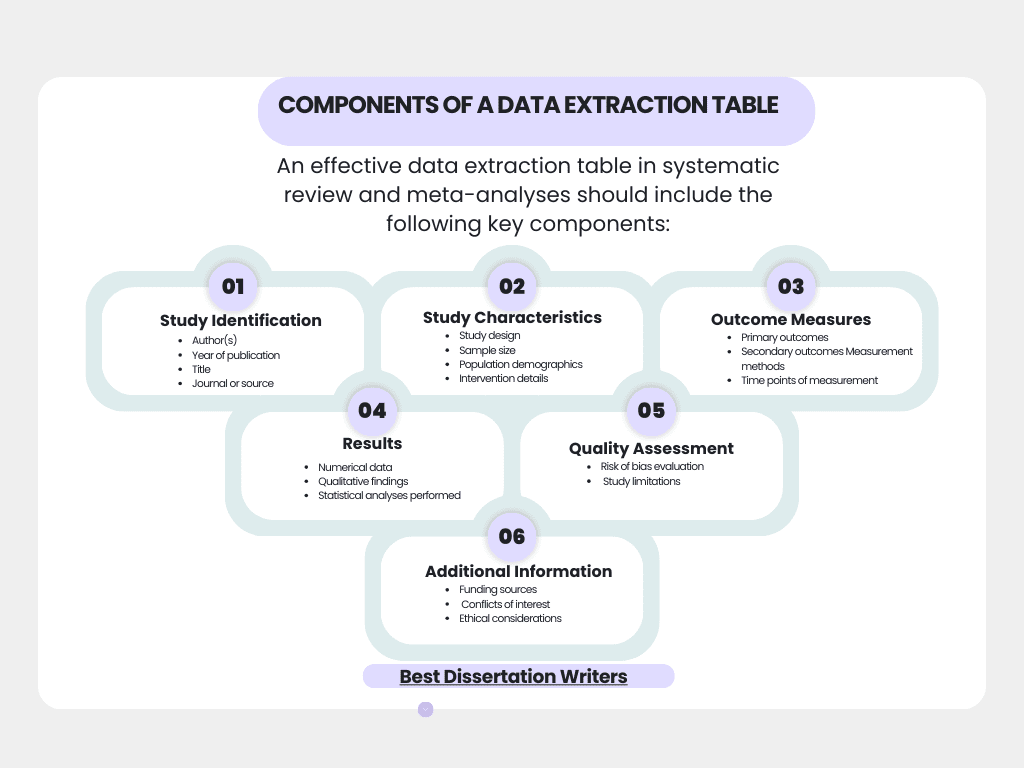
1. Study identification:
– Author(s)
– Year of publication
– Title
– Journal or source
2. Study characteristics:
– Study design
– Sample size
– Population demographics
– Intervention details
– Comparison or control group information
3. Outcome measures:
– Primary outcomes
– Secondary outcomes
– Measurement methods
– Time points of measurement
4. Results:
– Numerical data (e.g., means, standard deviations, effect sizes)
– Qualitative findings
– Statistical analyses performed
5. Quality assessment:
– Risk of bias evaluation
– Study limitations
6. Additional information:
– Funding sources
– Conflicts of interest
– Ethical considerations
Templates for Data Extraction Forms
Several templates are available to help review authors create data extraction forms:
- Cochrane template: The Cochrane Handbook for Systematic Reviews of Interventions provides a comprehensive template that can be adapted for various types of reviews.
- PRISMA checklist: While primarily used for reporting, the PRISMA checklist can guide the creation of extraction templates.
- BMC Medical Research Methodology: This journal offers templates and guidelines for data extraction in systematic reviews.
- Systematic Review Toolbox: An online resource providing various tools and templates for systematic reviews, including data extraction forms.
- Custom templates: Review teams often create custom extraction forms tailored to their specific research questions and review objectives.
Best Practices for Designing Extraction Tables
To ensure the effectiveness of your data extraction table, consider the following best practices:
- Pilot testing: Conduct a pilot test of the extraction form using a sample of included studies to identify any issues or missing elements.
- Clear instructions: Provide detailed guidelines for data extractors to ensure consistency in data collection.
- Flexibility: Allow for the addition of new data fields if unexpected information emerges during the extraction process.
- Use of categorical variables: Where possible, use predefined categories to facilitate data analysis and reduce ambiguity.
- Inclusion of free-text fields: Provide space for extractors to add notes or clarifications when necessary.
- Regular review: Periodically review the extraction process and make adjustments as needed to improve efficiency and accuracy.
- Dual extraction: Implement a system for independent extraction by two reviewers to minimise errors and bias.
By following these best practices, review authors can create robust data extraction tables that support high-quality systematic reviews and meta-analyses.
Nursing Dissertation Writing Help
Navigate the complexities of nursing research effortlessly with our expert systematic review and dissertation assistance. Our writers ensure your work shines with clarity and depth. Invest in your future—connect with Best Dissertation Writers today.
Example of Literature Matrix/Data Extraction Matrix/Evidence Table
With the research question of a nursing systematic literature review which states:
“Among patients with type 2 diabetes mellitus, what is the effectiveness of nurse-led educational interventions compared to standard care in improving self-management behaviors and glycemic control?” The following is an example of a complete literature matrix;
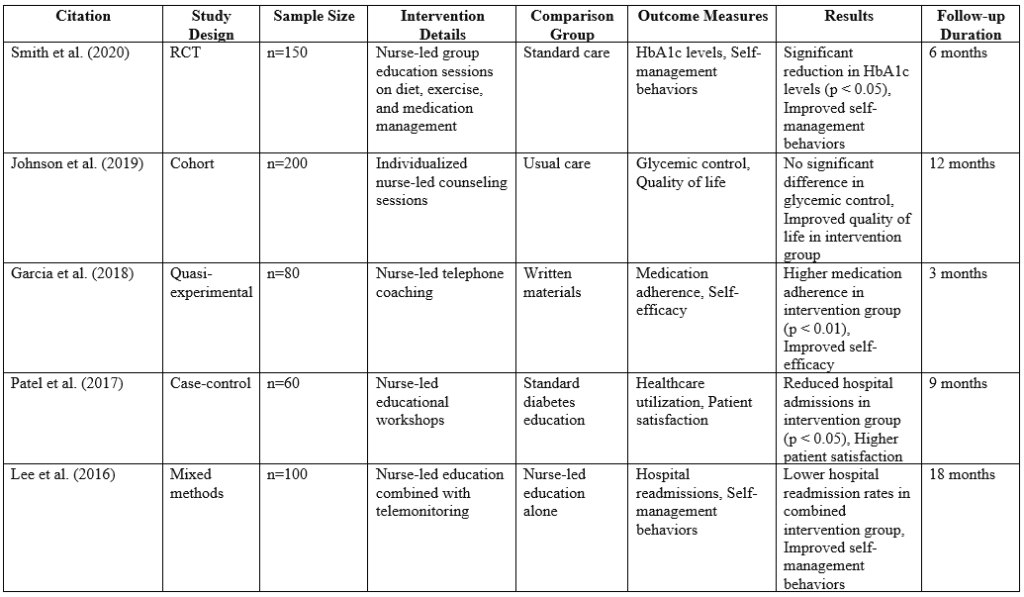
The table shows an example of a simplified literature matrix table for a systematic literature review on the effectiveness of nurse-led educational interventions compared to standard care in improving self-management behaviors and glycemic control among patients with type 2 diabetes mellitus. Please note that this example includes only a subset of potential data elements for illustration purposes, and you may need to customize the table further based on your specific research question and inclusion/exclusion criteria.
What Tools Are Available for Data Extraction?
Review of Data Extraction Tools
Several tools are available to assist with data extraction in systematic reviews:
- Covidence: A web-based platform that supports the entire systematic review process, including data extraction.
- EPPI-Reviewer: A comprehensive tool for all types of systematic reviews, offering features for data extraction and management.
- RevMan: Developed by Cochrane, this software includes data extraction capabilities alongside other review management features.
- DistillerSR: A versatile platform that supports various types of reviews and includes customisable extraction forms.
- Systematic Review Data Repository (SRDR): A free, web-based tool for extracting and managing data for systematic reviews.
- Microsoft Excel: While not specifically designed for systematic reviews, Excel remains a popular choice for creating data extraction tables.
Comparing Manual vs. Automated Data Extraction Tools
Manual Data Extraction:
– Pros:
– Greater control over the extraction process
– Ability to handle complex or nuanced data
– No need for specialised software
– Cons:
– Time-consuming
– Higher risk of human error
– Challenging to manage large volumes of data
Automated Data Extraction:
– Pros:
– Faster processing of large datasets
– Reduced risk of data entry errors
– Easier to standardise across multiple extractors
– Cons:
– May miss contextual nuances
– Requires initial setup and training
– Potential for systematic errors if not properly configured
The choice between manual and automated extraction often depends on the review’s scope, resources, and complexity of the data being extracted.
Using Excel for Data Extraction
Microsoft Excel remains a popular tool for data extraction in systematic reviews due to its flexibility and widespread availability. Here are some tips for using Excel effectively:
- Create a structured template with clearly labelled columns for each data point.
- Use data validation features to create drop-down lists for categorical variables.
- Implement formulas to automatically calculate summary statistics or effect sizes.
- Use conditional formatting to highlight missing data or potential inconsistencies.
- Create separate sheets for different types of data (e.g., study characteristics, outcomes, quality assessment).
- Use Excel’s filtering and sorting capabilities to easily review and analyse extracted data.
- Regularly save and backup your extraction file to prevent data loss.
While Excel is versatile, it’s important to note that more specialised tools may offer additional features specifically designed for systematic reviews.
Transform your nursing career with professional systematic review and dissertation support. Our skilled writers blend research expertise with academic precision to deliver outstanding results. Take the first step towards excellence—reach out to Best Dissertation Writers now.
What Data Should Be Collected in a Systematic Review?
Types of Data to Extract from Included Studies
When conducting a systematic review, it’s crucial to extract a comprehensive set of data from each included study. The types of data to collect typically include:
1. Bibliographic information:
– Authors
– Publication year
– Title
– Journal or source
2. Study characteristics:
– Study design (e.g., RCT, cohort, case-control)
– Setting (e.g., country, healthcare context)
– Sample size
– Duration of follow-up
3. Participant information:
– Demographics (e.g., age, gender, ethnicity)
– Inclusion/exclusion criteria
– Baseline characteristics
4. Intervention details:
– Type of intervention
– Duration and frequency
– Delivery method
– Comparator or control group information
5. Outcome measures:
– Primary and secondary outcomes
– Measurement tools or scales used
– Time points of measurement
6. Results:
– Effect sizes
– Confidence intervals
– P-values
– Subgroup analyses
7. Quality assessment:
– Risk of bias evaluation
– Study limitations
– Funding sources and conflicts of interest
How to Identify Relevant Data Points
To identify relevant data points for extraction, consider the following steps:
- Review your research question and objectives to ensure all necessary information is captured.
- Consult existing systematic reviews on your topic to see what data they collected.
- Involve content experts in the review team to identify critical variables in the field.
- Pilot test your extraction form on a sample of studies to identify any missing or superfluous data points.
- Be prepared to iteratively refine your data extraction table as you become more familiar with the literature.
Data to Collect for Meta-Analysis
If you plan to conduct a meta-analysis as part of your systematic review, additional data points may be necessary:
1. Detailed outcome data:
– Means and standard deviations for continuous outcomes
– Event counts for dichotomous outcomes
– Hazard ratios for time-to-event data
2. Sample sizes for each group
3. Statistical information:
– Test statistics (e.g., t-values, F-values)
– Exact p-values when available
4. Information on study design and analysis methods:
– Randomisation procedures
– Blinding methods
– Intention-to-treat or per-protocol analysis
5. Measures of heterogeneity within studies
6. Data for subgroup analyses or meta-regression
By carefully considering and collecting these data points, review authors can ensure they have the necessary information for a comprehensive systematic review and meta-analysis.
What Are the Common Challenges in Data Extraction?
Data Entry Errors and How to Avoid Them
Data entry errors can significantly impact the quality and reliability of a systematic review. Common types of errors include:
- Typographical errors: Typographical errors, often called typos, are mistakes made when typing or inputting data. These are usually unintentional spelling errors or incorrect keystrokes.
- Misinterpretation of data: Misinterpretation of data occurs when information is incorrectly understood or analyzed. It involves drawing incorrect conclusions from the available data due to errors in judgment or understanding.
- Incorrect unit conversions: Incorrect unit conversions happen when measurements are improperly changed from one unit to another. For example, mistakenly converting kilometers to miles using the wrong conversion factor.
- Transposition of numbers: Transposition of numbers is the accidental reversal of digits when recording or copying numbers. For instance, writing 53 instead of 35.
- Missing data points: Missing data points refer to gaps in a dataset where expected information is absent. This can occur due to various reasons such as incomplete reporting, data loss, or failure to collect certain information.
To minimise these errors:
- Implement dual data extraction with independent reviewers
- Use standardised data extraction forms with clear instructions
- Conduct regular quality checks and data verification
- Utilise automated data validation tools where possible
- Provide thorough training for data extractors
- Pilot test the extraction process before full implementation
Dealing with Incomplete Data
Incomplete data is a common challenge in systematic reviews. Strategies to address this issue include:
- Contacting study authors for missing information
- Using statistical methods to impute missing data when appropriate
- Clearly documenting missing data in the extraction table
- Conducting sensitivity analyses to assess the impact of missing data
- Considering the use of ‘unclear’ or ‘not reported’ categories in the extraction form
- Discussing the implications of missing data in the review’s limitations section
Managing the Review Process and Team Dynamics
Effective management of the review process and team dynamics is crucial for successful data extraction:
1. Clear role definition:
– Assign specific responsibilities to each team member
– Establish a chain of communication for addressing issues
2. Regular team meetings:
– Discuss progress and challenges
– Ensure consistency in interpretation of data
3. Standardised training:
– Provide comprehensive training on the extraction process
– Conduct periodic refresher sessions
4. Quality control measures:
– Implement a system for reviewing extractions for consensus
– Regularly assess inter-rater reliability
5. Time management:
– Set realistic deadlines for each stage of the extraction process
– Use project management tools to track progress
6. Conflict resolution:
– Establish a protocol for resolving disagreements in data extraction
– Involve a third reviewer or content expert when necessary
By addressing these common challenges, review teams can enhance the accuracy and efficiency of the data extraction process, ultimately improving the quality of their systematic review.
How Do Data Extraction Methods Impact Systematic Reviews?
Overview of Different Data Extraction Methods
Various data extraction methods can be employed in systematic reviews, each with its own impact on the review process:
1. Manual extraction:
– Traditional method involving human extractors
– Suitable for complex data or small-scale reviews
2. Semi-automated extraction:
– Combines human judgment with software assistance
– Can increase efficiency while maintaining accuracy
3. Fully automated extraction:
– Uses machine learning and natural language processing
– Potentially faster but may miss nuanced information
4. Crowdsourcing:
– Involves distributing extraction tasks to a large group of individuals
– Can be efficient but requires careful quality control
5. Hybrid approaches:
– Combines multiple methods to balance efficiency and accuracy
Impact of Extraction Methods on Data Analysis
The choice of extraction method can significantly influence data analysis in systematic reviews:
1. Data quality:
– Manual methods may provide higher accuracy for complex data
– Automated methods can reduce human error but may miss contextual nuances
2. Consistency:
– Automated methods can ensure greater consistency across large datasets
– Manual methods may introduce variability between extractors
3. Comprehensiveness:
– Manual extraction may capture more detailed information
– Automated methods might miss data not explicitly stated in standardised formats
4. Time and resources:
– Automated methods can significantly reduce time and labour costs
– Manual methods often require more time and human resources
5. Scalability:
– Automated methods are more easily scalable for large reviews
– Manual methods may become impractical for very large datasets
Elevate your nursing research with expert systematic review writing assistance. Our seasoned dissertation writers will guide you through the process, ensuring a comprehensive and impactful review. Don’t let complexity hinder your academic progress—reach out to us today.
Choosing the Right Method for Your Review
Selecting the appropriate extraction method depends on several factors:
1. Review scope and size:
– Larger reviews may benefit from automated or semi-automated methods
– Smaller, more focused reviews might be suited to manual extraction
2. Data complexity:
– Highly nuanced or context-dependent data may require manual extraction
– Standardised, quantitative data might be suitable for automation
3. Available resources:
– Consider the time, budget, and expertise available to the review team
4. Type of studies included:
– Reviews of interventions may have more structured data suitable for automation
– Qualitative reviews often require manual extraction to capture nuanced findings
5. Technological capabilities:
– Assess the team’s ability to implement and manage automated tools
6. Quality requirements:
– Consider the level of precision required for the review’s objectives
7. Reporting guidelines:
– Ensure the chosen method aligns with relevant reporting guidelines for systematic reviews
By carefully considering these factors, review authors can select the most appropriate data extraction method to enhance the quality and efficiency of their systematic review. The impact of data extraction methods on systematic reviews is significant, influencing not only the process but also the final results and conclusions drawn from the review.
Summary
A data extraction matrix or table in a systematic literature review and meta-analysis is a structured framework used to organize and synthesize information extracted from included studies. It typically consists of rows representing individual studies and columns representing key categories such as study design, sample size, intervention details, comparator, outcome measures, results, quality assessment, and notes/comments. The matrix facilitates systematic recording and comparison of study characteristics and findings, enabling researchers to analyze and synthesize evidence effectively.
It promotes consistency, transparency, and reproducibility in the review process by providing a standardized format for documenting extracted data. Additionally, the data extraction matrix serves as a valuable tool for quality assessment, facilitating the evaluation of study quality and risk of bias. Overall, the matrix plays a crucial role in synthesizing evidence from multiple studies to inform evidence-based practice and decision-making.
FAQs about Data Extraction Tables
What are data extraction tables?
Data extraction tables are essential tools used within systematic reviews in health research and literature reviews. These tables serve as a structured framework for the extraction of data from included studies. Review teams use these tables to systematically collect and organize relevant information throughout the review process. The data extraction template typically contains text fields for recording study characteristics and results.
These tables are crucial for data extraction and synthesis, ensuring consistency across the review team on the extraction categories. They can be adapted from one review to the next, depending on the specific needs of each systematic review. Data extraction tables are fundamental to the systematic review process, facilitating the availability of data for analysis and reporting later in the review.
What is an example of data extraction?
An example of data extraction in a systematic review of health interventions might involve using a standardized data extraction template with text fields to collect information from each study. The review team would extract data related to study characteristics, such as sample size, participant demographics, and intervention details. They would also record outcome measures and results. This process ensures that all relevant data is systematically captured.
For instance, in a review of a new drug therapy, extractors might record dosage, duration of treatment, adverse effects, and efficacy measures. The choice of data to extract would be determined by what is relevant to the review question. This systematic approach to data extraction helps prevent data entry errors and ensures consistency across studies.
How to do a data extraction?
To perform data extraction in a systematic review, start by creating and publishing a data extraction template tailored to your research question. Train data extractors on using the extraction form to ensure consistency. Begin the data extraction step by systematically reviewing each included study, entering relevant data into the template. This process involves careful screening of the full text, extracting information on study characteristics, methods, participants, interventions, outcomes, and results.
Use existing systematic reviews as a guide for what data will be required. Implement a system for dual extraction, where two reviewers independently extract data to minimize errors. Regular team meetings can help resolve discrepancies. Throughout the process, be prepared to refine the extraction categories as needed based on the studies encountered.
What is data extraction in Excel?
Data extraction in Excel is a common method used in systematic reviews and health research. It involves using Excel spreadsheets as a data extraction template, with text fields for different data categories. This approach is popular due to Excel’s widespread availability and flexibility. Reviewers can create custom extraction forms with multiple sheets for different types of data. Excel’s features, such as data validation and dropdown menus, can help prevent data entry errors and standardize responses.
However, more sophisticated systematic review automation tools are increasingly being used alongside or instead of Excel. These tools often offer more advanced features for data extraction and synthesis, such as automated or semi-automated data extraction capabilities. Nonetheless, Excel remains a valuable tool for many users of systematic reviews, especially for smaller projects or teams with limited resources.