What is a Stratified Sample?
Understanding the Concept of Stratified Sample
Stratified sampling is a probability sampling method that involves dividing a population into smaller subgroups, called strata, before conducting the sampling process. This sophisticated approach to survey sampling ensures that different segments of a population are adequately represented in the final sample. Unlike a simple random sample, stratified sampling involves dividing the population into mutually exclusive groups based on specific characteristics.
The concept of stratified sampling is often employed when researchers need to study distinct subgroups within a larger population. For example, when studying customer satisfaction across different age groups, stratified sampling can be used to ensure proper representation of each age category in the final sample.
“Don’t let dissertation stress hold you back. Best Dissertation Writers offers expert guidance, thorough research, and timely delivery to help you achieve academic excellence. Take the first step toward your degree—contact us today!”
How is a Stratified Sample Different from a Random Sample?
While simple random sampling or systematic sampling treats the entire population as one unit, stratified sampling technique acknowledges and accounts for population heterogeneity. In simple random sampling, every member of the population has an equal chance of being selected, but this method doesn’t guarantee representation from all important subgroups.
The key differences include:
1. Organization: Stratified sampling involves dividing a population into distinct groups before sampling, while random sampling takes a random sample of the population without preliminary grouping.
2. Representation: Using a random sampling method might miss smaller but significant subgroups, whereas stratified sampling ensures representation from each stratum.
3. Precision: Stratified sampling can produce more precise estimates than simple sampling, especially when studying diverse populations.
4. Complexity: While simple random sampling method is straightforward, stratified sampling involves more complex procedures and planning.
How Does Stratified Random Sampling Work?
Steps Involved in Stratified Random Sampling
The stratified sampling process follows a systematic approach:
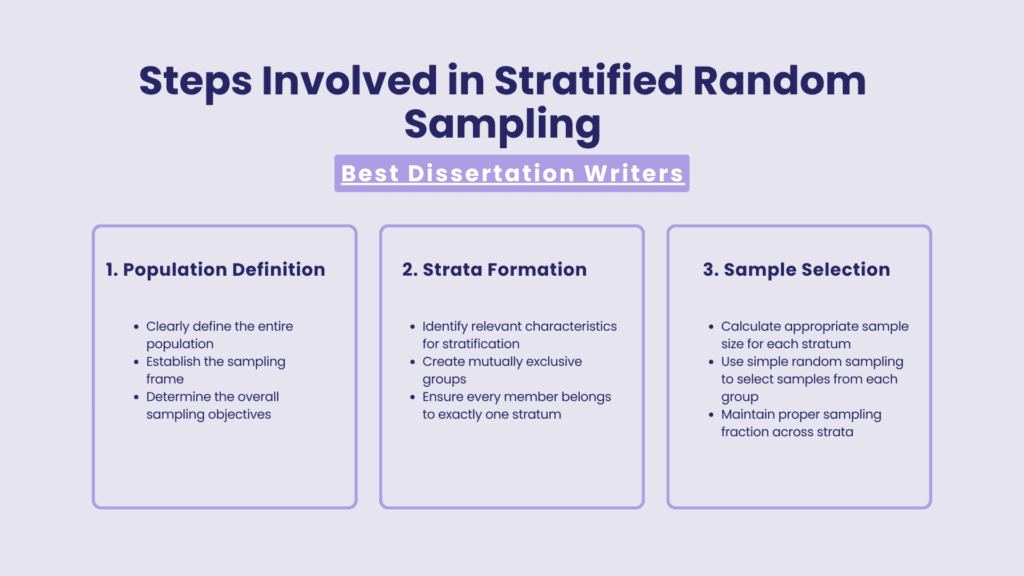
1. Population Definition:
– Clearly define the entire population
– Establish the sampling frame
– Determine the overall sampling objectives
2. Strata Formation:
– Identify relevant characteristics for stratification
– Create mutually exclusive groups
– Ensure every member belongs to exactly one stratum
3. Sample Selection:
– Calculate appropriate sample size for each stratum
– Use simple random sampling to select samples from each group
– Maintain proper sampling fraction across strata
How to Divide the Population into Strata?
When using disproportional stratified sampling or proportionate sampling, the division of population into strata must follow certain principles:
1. Homogeneity: Members within each stratum should share similar characteristics
2. Heterogeneity: Strata should be distinctly different from each other
3. Relevance: Stratification criteria should align with research objectives
Different sampling approaches may be needed depending on the population characteristics. The sampling rate for each stratum can vary based on research needs and population distribution.
Importance of Sample Size in Stratified Sampling
Sample size determination is crucial in stratified random sampling. When you take a random sample from each stratum, consider:
– Population size within each stratum
– Desired confidence level
– Acceptable margin of error
– Resource constraints
– Expected response rates
What Are the Advantages of Using Stratified Sampling?
Benefits of Stratified Random Sample in Research
Stratified sampling is a probability sampling technique that offers several key advantages:
1. Improved Precision:
– Reduces sampling error
– Provides more precise estimates
– Enables better population representation
2. Cost-Effectiveness:
– Sampling helps optimize resource allocation
– More efficient than simple random sampling or systematic sampling
– Better data quality per unit cost
3. Subgroup Analysis:
– Facilitates comparison between strata
– Enables detailed analysis of population segments
– Supports targeted research objectives
When to Use Stratified Sampling for Better Results?
Stratified sampling is used effectively in situations where:
1. Population is heterogeneous
2. Subgroup analysis is important
3. Precise estimates are needed
4. Cost constraints exist
5. Different sampling techniques might introduce bias
What Are the Disadvantages of Stratified Sampling?
Common Issues with Stratified Random Sampling
While stratified sampling is a method that offers many benefits, it also has limitations:
1. Complexity:
– Sampling involves more planning than simple random sampling
– Requires detailed population knowledge
– More complex analysis procedures
2. Resource Requirements:
– Higher initial setup costs
– More time-consuming than non-probability sampling methods
– Requires skilled researchers
3. Potential Issues:
– Sampling bias if strata are poorly defined
– Difficulty in achieving proper stratification
– Challenges in maintaining sampling frame accuracy
“Transform your research vision into reality with Best Dissertation Writers. Our team of PhD-qualified experts specializes in crafting exceptional dissertations across all disciplines. Start your journey to academic success—schedule your consultation now!”
How to Mitigate Disadvantages in Stratified Sampling?
To address these challenges:
1. Careful Planning:
– Thoroughly define strata
– Use appropriate sampling fraction
– Maintain clear documentation
2. Quality Control:
– Regular review of sampling process
– Validation of strata definitions
– Monitoring of sampling error
3. Resource Management:
– Efficient allocation of resources
– Training of research team
– Use of appropriate technology
Types of Stratified Random Sampling
Disproportionate vs. Proportionate Stratified Sampling
There are two main approaches to stratified sampling:
1. Proportional Stratified Random Sampling:
– Sample size proportional to stratum size
– Maintains population proportions
– Simpler to implement and analyze
2. Disproportionate Sampling:
– Varying sampling rates across strata
– Used when certain strata need more attention
– More complex but potentially more effective
Cluster Sampling and Its Relation to Stratified Sampling
While stratified sampling and cluster sampling are both probability sampling methods:
– Cluster sampling involves selecting groups instead of sampling individuals
– Stratified sampling ensures representation from all strata
– Different sampling approaches serve different research needs
How to Implement Stratified Sampling in Your Research?
Choosing the Right Method of Sampling for Your Study
When deciding whether to use stratified random sampling:
1. Evaluate Research Needs:
– Study objectives
– Population characteristics
– Resource availability
– Required precision
2. Compare with Alternatives:
– Simple random sampling or systematic sampling
– Quota sampling (non-probability sampling method)
– Convenience sample approaches
– Other sampling techniques
3. Consider Implementation Factors:
– Available resources
– Timeline constraints
– Technical capabilities
– Budget limitations
Tips for Effective Stratified Random Sampling
To ensure successful implementation:
1. Preparation Phase:
– Clearly define research objectives
– Identify appropriate stratification variables
– Develop comprehensive sampling frame
– Calculate required sample sizes
2. Implementation Strategies:
– Use appropriate random selection methods
– Maintain detailed documentation
– Monitor sampling process
– Implement quality control measures
3. Best Practices:
– Regular validation of strata definitions
– Continuous monitoring of sampling fraction
– Proper training of research team
– Regular review of sampling techniques
4. Common Pitfalls to Avoid:
– Overlapping strata
– Insufficient sample sizes
– Poor documentation
– Inadequate quality control
5. Quality Assurance:
– Regular audits of sampling process
– Validation of sample units
– Monitoring of response rates
– Assessment of sampling bias
“Struggling with your dissertation? Join thousands of successful graduates who trusted Best Dissertation Writers. Our proven methodology and dedicated support ensure your research stands out. Unlock your potential—reach out today!”
When properly implemented, stratified sampling becomes a powerful tool for researchers. Whether using proportional random sampling or disproportionate sampling, the key is to maintain rigorous adherence to sampling protocols while remaining flexible enough to address challenges as they arise.
The success of stratified sampling relies heavily on proper planning, execution, and monitoring. By following these guidelines and maintaining awareness of both advantages and disadvantages of stratified sampling, researchers can effectively utilize this method to achieve their research objectives.
FAQs about Stratified Sampling Method
What is meant by stratified sampling?
Stratified sampling method is a research technique that’s stratified, meaning it divides a population into smaller subgroups (strata) before samples are selected. This method of sampling that involves categorizing the population based on shared characteristics ensures each subgroup is represented proportionally. Probability sampling means that every member within each stratum has an equal chance of selection. After dividing the population into strata and take a random sample from each group, random samples are then selected. This approach ensures better representation than simple random sampling, especially when studying diverse populations.
Which is an example of a stratified sample?
Consider a university conducting sampling studies of student satisfaction. Instead of using quota random sampling or random or systematic sampling, they employ stratified sampling. For example, they first divide students by year (freshman, sophomore, junior, senior) and then select a sample from each group proportionally. This ensures representation across all years. Sampling isn’t just about picking random students; it’s about ensuring each academic year is properly represented. If the university has 1000 students with 250 per year, they might select 25 students from each year for a 10% sample.
What is stratified sampling best used for?
Stratified sampling is best used when you need to ensure specific subgroups are represented in your study. Sampling is that it allows researchers to capture data from all important segments of a population. Random sampling involves simple selection, but stratified sampling provides better precision when studying diverse groups. This approach is particularly valuable when include simple random sampling wouldn’t adequately represent smaller but significant subgroups. It’s ideal for populations with distinct characteristics where sampling does not require equal group sizes, such as market research across different income levels or age groups.
What is stratified vs random sample?
Random sampling is a method where sampling means that every member has an equal chance of selection, without considering subgroups. In contrast, stratified sampling first divides the population into distinct groups before sampling. While both are probability sampling techniques, they differ in their approach to selection. In simple random sampling, you might miss important subgroups, but stratified sampling ensures representation from each stratum. Think of it as the difference between randomly picking names from a hat versus organizing names into categories first and then selecting from each category.